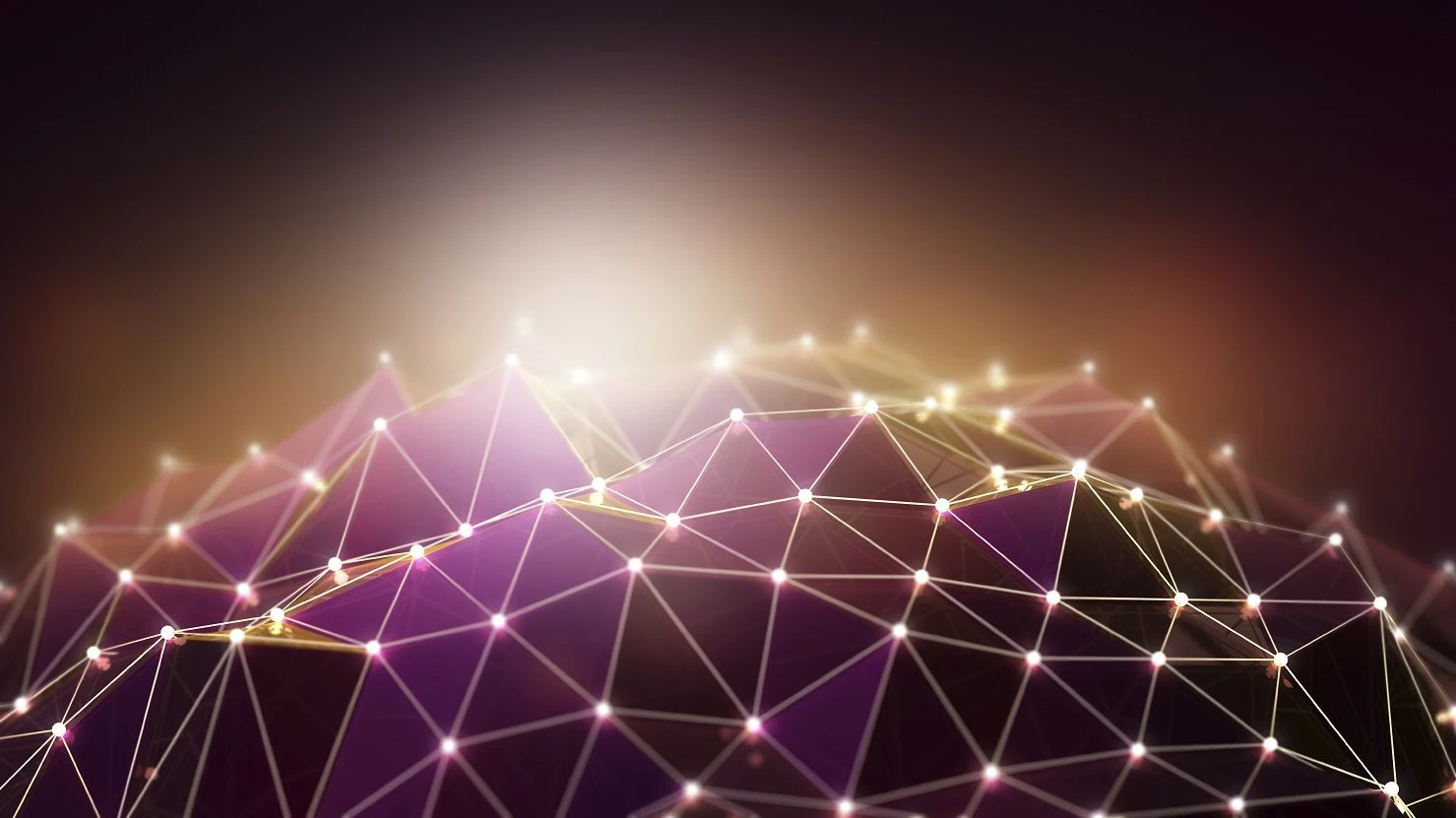
Member Article
The new predictive analytics: The democratisation of insight
In old-school boardroom meetings, executives brainstormed about the next new thing, using only sales charts and intuition. Today, predictive analytics tools take the guesswork out of predicting what customers will want and need in the future. Until recently, only large, established companies like Google or Amazon.com could afford predictive analytics on big data. But a generation of new tools is driving the democratisation of predictive analytics by enabling enterprise developers to build their own analytics applications.
PA to the people: The evolution of predictive analytics
Today’s predictive analytics incorporates machine learning on big data, but the traditional version of predictive analytics has been around for quite some time, with limited adoption.
Traditional predictive analytics, represented by statistical tools and rules-based systems (previously known as expert systems) is stuck in the 1990s. It’s based on data in relational data warehouses, which handle only structured data collected in batches. Signals from real-time data, such as the location of a mobile phone or signals available in social data such as tweets or customer service text data, are not considered.
Further, many of the tools out there are severely limited by scale and only handle data that fits in memory, which forces analysts to work with samples rather than the full data. Sampling captures the strongest signals in data but misses out on the long tail of weaker signals, thus giving up a fair amount of precision.
Traditional predictive analytics also requires feature design: an analyst manually designs the features that drive predictions through a hypothesize-and-test process. For example, for predicting retail purchases, the analyst might hypothesize three features: total amount spent by the customer in the past, total number of times the customer has purchased in the last year, and the last date they made a purchase. The analyst then tests which of these features carry predictive power and experiments with various predictive algorithms.
Finally, traditional predictive analytics fails to adapt when customer behaviour changes. Predictive models are typically implemented as code inside applications, which makes it impossible to even monitor their performance, much less adapt to change.
The world is moving too fast, and consumers change behaviour too quickly, for traditional predictive analytics to keep up. But a major advance has pushed predictive analytics past these hurdles: machine learning.
Machine learning: Giant innovation
Over the past decade, internet giants like Google, Yahoo!, and Amazon figured out that using big data enabled them to serve customers better. These companies drove innovation in ways that made predictive analytics more precise and more affordable. Their respective successes are the proof points for deriving competitive advantages through predictive analytics on big data; this is now driving many enterprises to employ their data to increase competitiveness.
Three advances that have made predictive analytics both more affordable and more precise: machine learning; distributed data processing; and plummeting hardware costs.
As we hinted at earlier, the most important advance is machine learning, an artificial intelligence technology that permits computers to adaptively learn from big data without requiring further programming. For example, machine learning can help a telecom company precisely predict which customers are likely to churn when their contract expires. It does so by analysing their usage and billing patterns alongside customers’ sentiment, pulled from call notes taken by customer service representatives. Machine learning has put us on the path of increasing automation in feature design, which is a fundamental advance.
Machine learning at scale requires marrying it with distributed data processing technology. Hadoop is the modern distributed data processing technology that scales on commodity hardware. It enables companies to collect, store, and process big data more cost effectively than would have been feasible through older relational database management system technologies.
APIs: Driving predictive analytics adoption
We are seeing an increasing percentage of real-time customer interactions flow through application programming interfaces (APIs). Bringing together predictive analytics with APIs helps businesses produce predictive apps that know their customers, understand what the customer needs, and deliver to the customer what they want, before they know they want it.
This makes APIs a great place both to collect data as well as expose real-time predictive analytics to app developers. At the end of the day, it’s the usage of predictive analytics that drives business value; we think APIs are the way to drive adoption of real-time predictive analytics.
This was posted in Bdaily's Members' News section by Apigee .