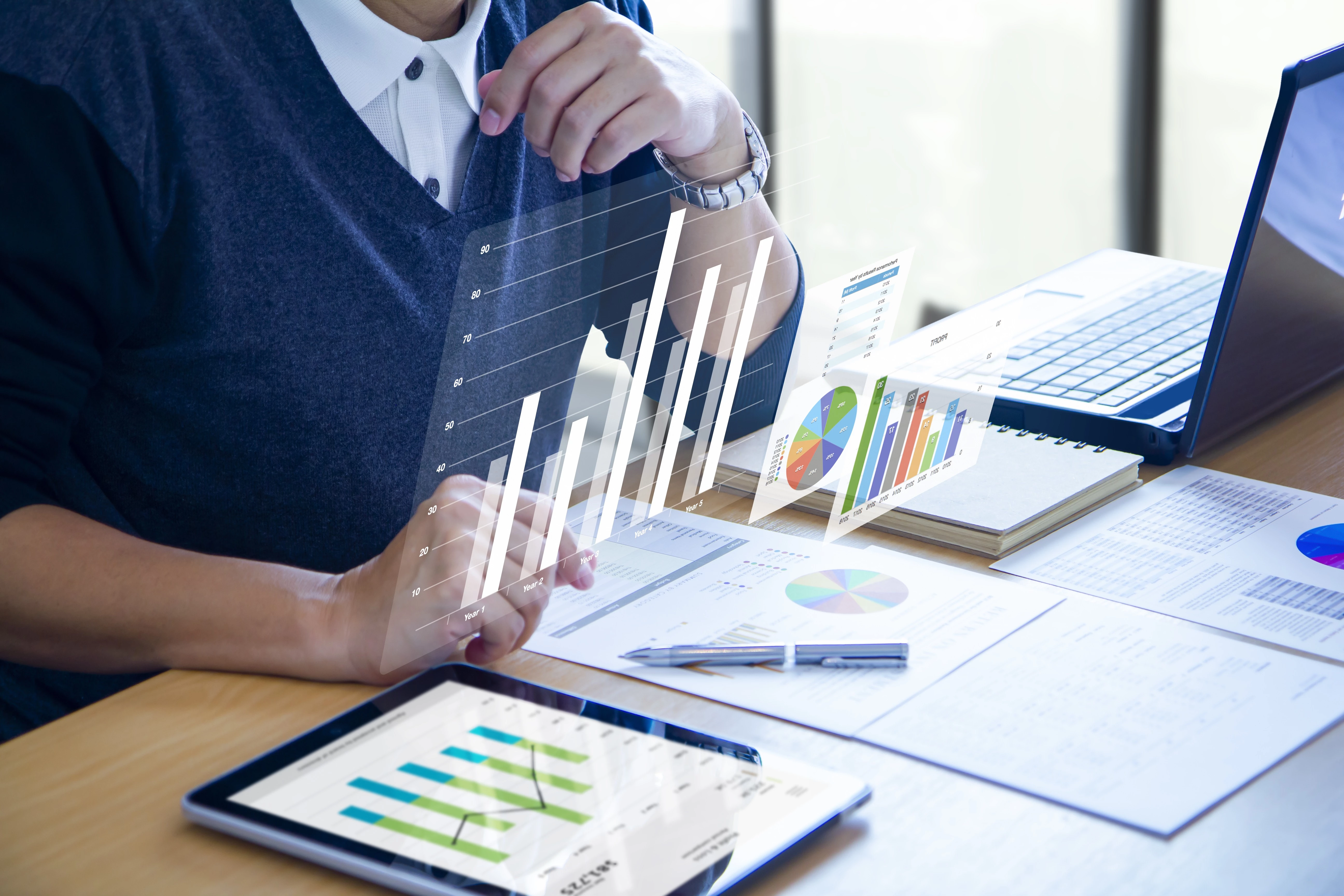
Partner Article
Is Synthetic Data the hoped-for game changer for Artificial Intelligence?
Artificial intelligence (AI) and machine learning (ML) are immensely data hungry in their training and development. Collecting and labelling these enormous datasets with thousands or even millions of objects is time consuming and costly. In addition, over the last few years, there has been increasing concern about how biased datasets can lead to AI algorithms perpetuating systemic discrimination. As far back as 2018 Gartner predicted that 85% of AI projects up to 2022 would deliver unsatisfactory outcomes due to bias in data, algorithms or the teams responsible for managing them. Synthetic data offers a potential solution to the dilemmas of time, cost and bias. However, two big questions are often raised: How effective is synthetic data? And can it fully or partially replace real data to solve real problems?
Synthetic data refers to a range of computer-generated data types that simulate original, real-life records. It can be created by stripping any personally identifiable information from a genuine dataset to fully anonymise it; or the original dataset can be used as the basis for a generative model that produces highly realistic data values and qualities. It aligns well with the data-centric approach in applications such as optical character recognition (OCR), natural language processing (NLP) and speech & language processing (SLP). Mastering these tasks helps in building reliable solutions for multiple real-world problems - from solving a specific task such as fruit classification to building virtual assistants which can process information from a variety of sources and modalities. However, as the architectures of AI become more complex, AI remains only as good as the data it is trained on. This is where we see the bottlenecks in AI: There is always a need for large and diverse datasets to advance AI models.
Synthesising in the real world
A ready example can be seen in speech processing, where the two fundamental tasks are speech recognition and speech synthesis. Speech recognition, also known as Speech-to-Text, is a technology that allows a program to convert human speech into written text, whereas speech synthesis attempts to synthesise natural and comprehensible speech from input text. In both scenarios, a considerable amount of data, specifically transcripts and accompanying speeches, is necessary to create the programs. Languages possess biases such as accent sensitivity and multi-dialect, however, it is not always straightforward to collect data with these variants. This is where synthetic data can show its great potential; with small amounts of samples in target accents, a great amount of data can be generated to boost performance of the systems and cover a wider range of vocabularies, words, dialects, and languages. Similarly, a speech recognition model is expected to recognize almost all voices, regardless of different human biases, including vocalisations, articulations and pronunciations.
Synthetic data is also important in real-time applications with limited labels for specialised domains such as insurance, healthcare, banking etc. When a client asked for a model to be built with just 755 short text examples, this was clearly insufficient. However, when the sample was used to generate synthetic data to teach the AI model, the results show that model accuracy increased from 64% to 89%, surpassing the customer’s performance requirements.
Bias tends to creep into artificially generated datasets because it accurately mimics and can therefore reproduce or even amplify any bias inherent in the original data. Bias can occur due to where, when and how the original dataset was collected and with what purpose. If the original data was collected from students or a self-selecting group of applicants for, say, loans or respondents to questions on a website, they may not be representative of the general population. Age, race, gender, socio-economic grouping, marital status and many other biases can be over- or underrepresented in the original dataset. We have come a long way compared to the past. Bias in AI and ML is when the model favours certain predictions/conditions over others. Extensive research has been carried out to help detect and mitigate bias. Data scientists must train the AI and ML models to account for bias and ensure the synthetic dataset delivers impartiality.
Fuelling future evolution
Data synthesis is essential for improving the quality and quantity of robust training data for advanced algorithms and models. Synthetic data presents in all types of modalities, from textual documents to images, videos, and tabular data. They all play an important role in corresponding applications. Each wave of AI innovation builds on the previous generation.
Research to develop autonomous gadgets such as robots, drones and self-driving car simulations pioneered the use of synthetic data. This is because real-life testing of robotic systems is expensive and slow. Synthetic data enables companies to perform product testing in thousands of simulated scenarios at lower cost. As synthetic data enables training AI systems in a completely virtual realm, it can be readily customised for various uses ranging from healthcare and automotive to financial services. It has the potential to fuel leaps forward in many sectors. Synthetic patient and customer data can improve machine learning/deep learning model accuracy by increasing the training dataset size exponentially without violating data privacy regulations. With synthetic data, medical diagnostics and, for example, fraud detection methods can be tested and evaluated at scale for their effectiveness.
Realising the full potential of AI
In the best scenario, real-world data is always the first choice for any AI based solution. However, it is difficult to get real-world data due to the constraints of privacy and cost. Synthetic data is the best alternative, though generating realistic synthetic data is not an easy task. Synthetic data can be generated at large scale and it is much more cost effective compared to real-world data. As the elimination of bias is better achieved, synthetic data can deliver a better real-world simulation to develop insights and test hypotheses quickly. With low costs and speed of delivery, it is a game changer, enabling the full potential of AI and ML to be deployed across a wide range of industries.
This was posted in Bdaily's Members' News section by Julia Vockrodt .