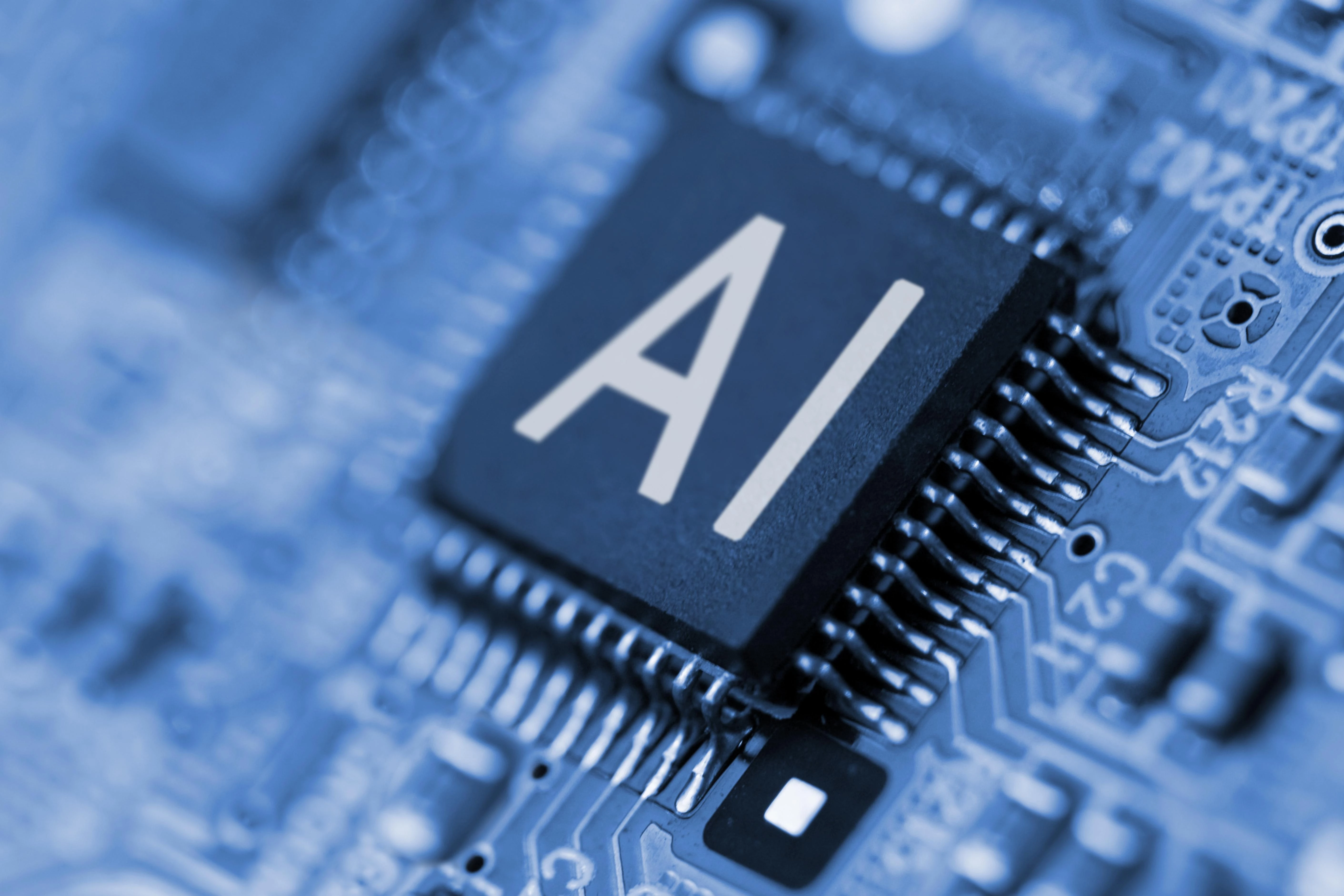
Partner Article
Sounds Good - AI to Revolutionise the Analysis of Acoustic Data
Acoustic quality inspection of machinery, equipment and products with 95% accuracy Acoustic monitoring for predictive maintenance gives early warnings of potential problems
We have all seen the antiques expert flick a fingernail against a piece of porcelain or glass to see if it ‘rings true’ or returns a flat note indicating a hairline crack somewhere in the item being examined. Sound is an important tool in detecting faults or problems from machines to engines, weld integrity to ball-bearing races and a wide range of products. Whereas visual inspection has substantially been taken over by sensors, sound has remained the province of the human ear in most cases. The problem has been that the frequency of equipment failure in real environments is low and the number of ways in which equipment can fail is large; an expert can hear the difference, but the ear can be distracted by other noises or overwhelmed by a noisy environment. An obvious answer is to use an electronic listening device to pick out anomalies but if anomalies are few and far between the training of such a device would be very slow, very expensive and specific to individual applications.
In Manufacturing, valuable assets are often expensive, difficult to replace and costly in terms of associated lost production. It is crucial for business operation to ensure the maximum lifespan and optimal condition of machinery, equipment, and production lines. Previously, the interpretation of sounds in industrial settings was the confine of a few experienced maintenance and operations personnel. The advent of AI has revolutionised the analysis of acoustic data, providing a new source of information that can be utilized to solve real-world problems.
The challenge to create a solution that would identify anomalies indicating faults in the sound produced by a wide range of machines, equipment and products was put to the artificial intelligence (AI) team at FPT Software. Conventional approaches to the problems had failed to produce acceptably high levels of detection. So, they looked for a new way of solving the problem. The solution they developed uses a mixture of different sound representations, a Mixed Feature, and a new auto-encoder architecture, a deep-learning method called a Fully Connected U-net (FCUN) to increase the resolution of the output. AI can automatically analyse, quantify, tag and identify the source of sounds at a scale and level of precision that was previously unimaginable.
AI, coupled to machine learning (ML), is then used to learn the representation of normal data and detects abnormal data when discrepancies are detected between normal data and new data. Trained with normal data it identifies potential issues, before they result in downtime or production delays, with an accuracy of 95%. A single machine-learning model for different types of sound helps to simplify installation and increase predictive latency in real time. Deployment in many applications, to start achieving working results, is often as quick as two weeks. The result is a machine learning operations platform (MLOps) that uses acoustic anomaly detection to automate product and machine inspection and health monitoring as well as providing predictive maintenance data for equipment.
FPT Software’s acoustic anomaly detection product, SoundAI, won the top award for Innovation in Product Design and Development in its class in the Stevie Awards, one of the world’s most coveted prizes made in recognition of the achievements and positive contributions of organisations and working professionals worldwide. The platform can easily be deployed to any edge devices with low-code requirements from the customer. The result supports machine health monitoring, even with no abnormal data, improves accuracy continuously with a detection rate of above 95%.
Dr Phong Nguyen, chief AI officer at FPT Software added: “Sound can play a vital role in the predictive maintenance of manufacturing equipment, as unusual or unexpected sounds from the equipment can be an early predictable indicator of potential failures, which may not be detected by visual inspection due to the complexity of an assembled machine. Sound sensors can be deployed without physical contact, which makes them suitable for applications where contact-based sensors may cause damage or be impractical.”
Over the past six months, SoundAI has been applied across industries such as manufacturing, healthcare, smart cities, smart house and household electronics. SoundAI complements FPT Software’s suite of inspection tools covering vision, sound, and sensor-based analysis, for manufacturers to effectively monitor, maintain and improve the quality of their products.
This was posted in Bdaily's Members' News section by FPT .