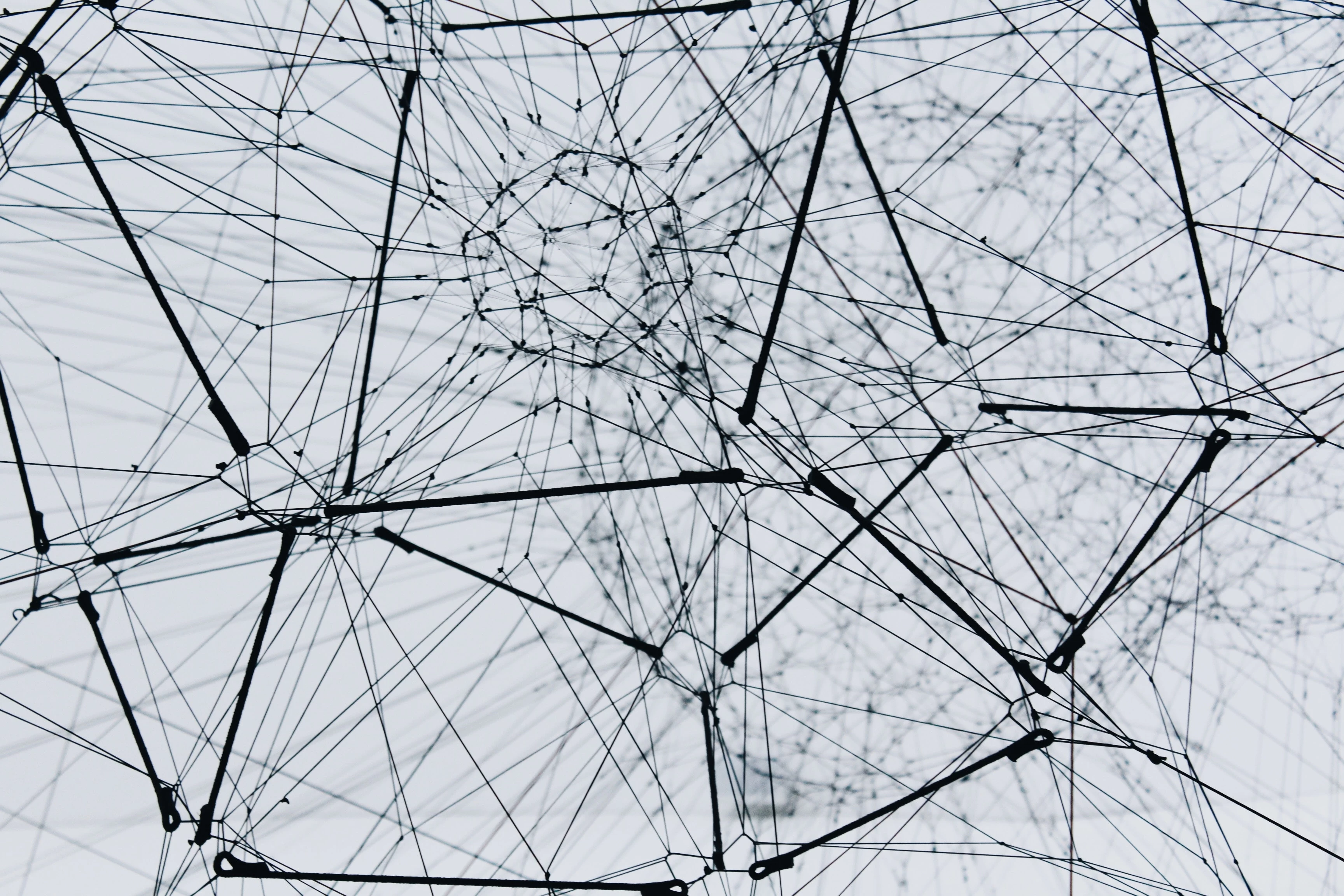
Member Article
Navigating the uncharted waters of generative AI - a data governance imperative
By Reece Griffiths, CEO & Co-founder of Deasie
The adoption of generative AI (GenAI) models is now a strategic priority for companies across the globe. However, as organizations eagerly dive into the world of GenAI, a common issue emerges: data readiness.
Data readiness: the missing piece
A significant contributor to the data readiness challenge is the absence of governance surrounding unstructured data. Historically, data governance has primarily focused on structured data (e.g., tabular datasets), leaving unstructured data—estimated to comprise 80% of all data by 2025 according to IDC—largely neglected.
CIOs & CDOs have historically had little to no incentive to invest in the management and quality control of unstructured data assets such as contracts, market reports, email chains, policies etc. However, through GenAI, such data is quickly becoming the foundation for a growing list of applications ranging from chatbots to content generation engines. As this happens, the historic lack of data infrastructure is becoming a serious blocker.
A front-row seat to enterprise data challenges
We previously led the build of an ML-data governance product within McKinsey/QuantumBlack which we deployed with 10+ Fortune 500 companies. Over the course of our collective 10+ years working with the world’s leading companies on their data & AI initiatives, the most consistent challenge we’ve encountered is a lack of data governance.
At the beginning of 2023, these companies rushed to build their first GenAI use cases, only to be hit with a wave of data readiness challenges. The data required for such use cases typically exists as tens or hundreds of thousands of documents and files, spread across anything from Dropbox, to the cloud, to legacy systems and even an individual’s local desktop.
So why does data governance matter? Let’s use an analogy: Imagine a language model deployed on a few thousand input documents and tasked with retrieving a specific piece of information. This request is akin to sending someone into an enormous warehouse of disorganized packages and asking them to bring back a specific box. In the warehouse, boxes are piled chaotically, labels are missing or misleading, some boxes are completely out of date, many look nearly identical. As the volume of parcels grows, so too does the probability of returning a package that is not quite right. Now imagine the same scenario in which every box is carefully labeled with visible tags related to the parcel’s contents, date, owner, purpose and so on – clearly, the likelihood of retrieval error drops significantly. Large language models (LLMs) are highly intelligent, but in the absence of data governance, their performance is severely limited.
Challenges of GenAI with unstructured data
Directly attaching a large language model to a sea of unstructured data is creating three core challenges for the enterprise:
- Accuracy: GenAI model performance will suffer when fed irrelevant, outdated, or inconsistent data. One of the biggest challenges is data being taken out of context, which occurs in the absence of sufficient metadata.
- Security: The fear of exposing GenAI models to sensitive information (e.g., Personal Identifiable Information) looms large. Challenges also exist with managing data access across a company i.e., ensuring that certain individuals are not indirectly exposed to data via a model that they otherwise should not be able to see.
- Efficiency: Running GenAI models on large volumes of data is computationally expensive. When a significant proportion of such data is actually not relevant for the specific task, it is highly inefficient and results in unnecessary costs.
A call for data governance
For companies looking to leverage GenAI at scale, data governance for unstructured data is no longer a nice to have, but a necessity. To harness the true power of this technology and ensure safe, reliable, and efficient deployment, enterprises must invest in robust data governance practices.
This will require some form of intermediary layer that serves as a bridge between the mass of data spread across an enterprise, and the growing list of GenAI applications built on top of it. Only by first creating a robust data foundation will companies be able to ensure that the data fed into a given model is verified in terms of relevance, quality and safety. Our approach as a data governance platform for language models focuses on integrating intelligent metadata labeling with vector embeddings. Combining these allows us to make the best possible assessment of key data quality and safety dimensions, offering potential pathways for businesses to address the challenges of applying GenAI to unstructured data.
We believe that having a strong data foundation will be the most important criteria for businesses aiming to adopt and benefit from GenAI in a scalable, secure, and efficient manner - and they need to start thinking about this now.
This was posted in Bdaily's Members' News section by Deasie .